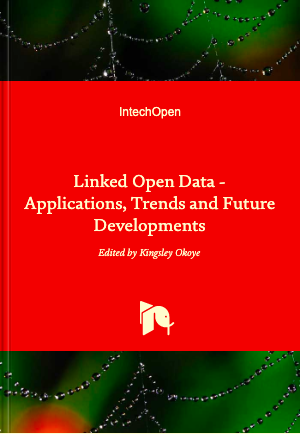
Linked Open Data – Applications, Trends and Future Developments
Nowadays, modern tools and techniques for the collection and analysis of data in all fields of Science and Technology are proving to be more complex. The growing complexities are evidenced by the need for a more generalized or precise description (integration) of the data sources and formats that allow for flexible exploration of the different data types. Perhaps, the challenge has been on how to create systems capable of providing an understandable format for different datasets, as well as, making the derived standards explicable across the different platforms. Over the past few decades, one of the recent technologies that have proved indispensable in this area is the Linked Open Data (LOD) cloud. The LOD consists of a number of machine-readable datasets with Resource Description Framework (RDF) triples that are useful in describing data classes and the properties. Moreover, indications from the early researches note that one of the problems with the existing data or information processing systems is the need for not just representing the data (or information) in formats that can be easily understood by humans, but also for building intelligent systems that trail to process the information that they contain or support. In other words, machine-understandable systems. By machine-understandable systems, we assume that the extracted information or models are either semantically labeled (annotated) to ease the analysis process, or represented in a formal structure (ontology) that allows a computer (the reasoning engine) to infer new facts by making use of the underlying relations. The main idea for any data or information processing system for those aspects of aggregating the data or computing the hierarchy of various process elements is that they should not only be machine-readable but also machine-understandable. Moreover, an adequate knowledge-based system is perceived to be, on the one hand, understandable by people, and on the other hand understandable by the machines. As devices become smarter and produce data about themselves, it will become increasingly important for scientists to take advantage of more powerful tools and/or data integration techniques to help provide a common standard for information dissemination across the different platforms. To this end, the content of this book shows that technologies such as the semantic web, machine learning, deep learning, natural language processing, and learning analytics which encompasses the wider spectrum of the Linked Open Data (LOD) are of paramount. Therefore, the work presents two main drivers for the Linked Open Data technologies: (i) encoding knowledge about specific data and process domains, and (ii) advanced reasoning and analysis of the big data at a more conceptual level. This book intends to provide the reader with a comprehensive overview of the current state-of-the-art within the Linked Open Data and the benefits of the methods – ranging from the semantics-aware techniques that exploit knowledge kept in (big) data to improve data reasoning (big analysis) beyond the possibilities offered by most traditional data mining techniques.
More details about the book project and submission of Chapters can be found at the following link.
Book Chapter Proposal and Submission link